I spent the last few weeks digging deeper into time series-related methods and data mining methods. For this post, I have decided to write a broad introduction related to the latter (data mining), since it may look more practical and also trendier than time-series (but watch out with the emergence of Particle Filtering and other Sequential Monte Carlo methods (SMC)).
Introduction to Data Mining and Criss Angel
So what is data mining? Data mining can be defined as the process but also the “art” of discovering/mining patterns, meaning and insights in large datasets by using statistical and computational methods. In other words, a data miner is like a Criss Angel (You can pick any other magician here!) that will make appear from your messy ocean of data, insights that will be valuable to your company and may give you a competitive advantage compared to your competitors; simply read Tom Davenport’s bestselling book “Competing on Analytics: The New Science of Winning” if you’re not convinced yet about the power of analytics and by extension of data mining. Furthermore, data mining related tasks are also considered as part of a more general process called Knowledge discovery in databases (KDD) which includes the “art” of collecting the right data as well as organizing and cleaning these data, which are also extremely important tasks prior to analyzing the data.
Some Brief History and a Link to Business Intelligence
Data mining mainly takes its roots from the fields of Statistics and Computer Science (some might say Artificial Intelligence) and may also be referred as “Statistical Learning”. From a statistical perspective, most early and recent advances coming from Statistics have come from the Stanford Statistics department school of thoughts (Leo Breiman (was at UC Berkeley), Bradley Efron, Jerome H. Friedman, Trevor Hastie and Robert Tibshirani). By the way, don’t forget that Stanford University is only 7 miles away from Google. Furthermore, the emerging field of Business Intelligence has blossomed as a combination of: (1) data mining tasks, (2) information systems technology and (3) crispy marketing insights.
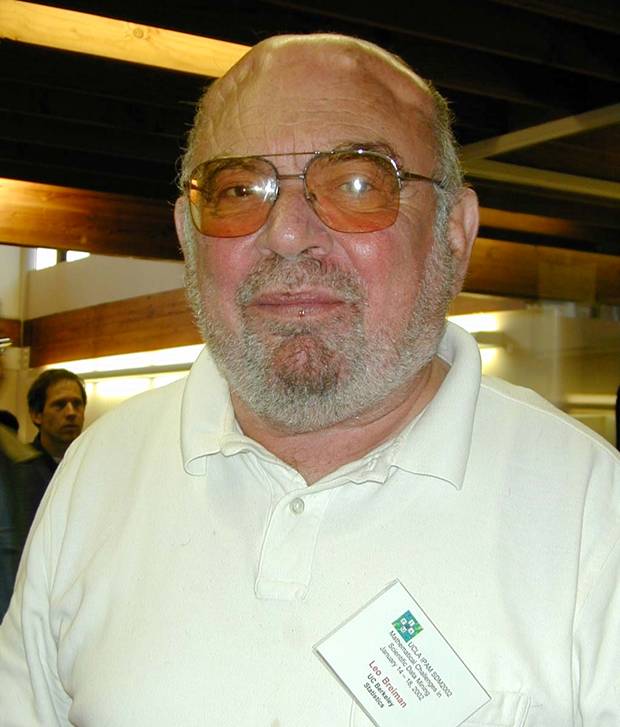
Types of Data Mining Methods and Marketing
Data mining methods can be divided in multiple ways. However, most books on the topic, and especially those related to marketing and business intelligence, will generally divide data mining methods into two types, the ones related to supervised learning and the ones related to unsupervised learning.
Supervised Learning
Supervised learning is often more associated to scientific research as it includes tasks where the data miner needs to describe or predict the relationship between a set of independent variables (also referred to as inputs, features) and a dependent variable (also referred to as outcome, output or a target variable). Moreover, the dependent variable can be categorical (i.e. churn rate or classes of customers) or continuous (i.e. money earned from that customer) while the independent variables may be of any type but needs to be coded properly (i.e. dividing the categorical variables into separate binary variables). From a marketing and business intelligence perspective, I will divide supervised learning into two interrelated tasks: (1) supervised classification tasks and (2) Predictive Analysis.
Supervised Classification tasks: Supervised classification tasks occurred when you want to predict correctly to which class/category (this is the dependent variable) belong the new observations (i.e. customers) based on results from an already known training dataset. Generally, you will achieve this task by using: (1) a training dataset, (2) a validation dataset and (3) a test dataset. Most known methods I’m using for these tasks are the following:
1. Multinomial Logit (MNL)
2. Linear Discriminant Analysis (LDA)
3. Quadratic Discriminant Analysis (QDA)
4. Flexible Discriminant Analysis with Multivariate Adaptive Regression Splines (FDA – MARS)
5. Penalized Discriminant Analysis (PDA)
6. Mixture Discriminant Analysis (MDA)
7. Naïve Bayes Classifier (NBC)
8. K-Nearest Neighbor (KNN)
9. Support Vector Machines with multiple Kernels (SVM)
10. Classification and Regression Trees (CART)
11. Bagging
12. Boosting
13. Random Forests
14. Neural Networks
Predictive Analysis: I’ve decided to include the expression “Predictive Analysis” here, since it’s a buzzword in the web community nowadays. However, any task related to supervised classification involve a so-called “Predictive Analysis”. However, “Predictive Analysis” is a broader expression that also includes tasks related to the prediction of a continuous dependent variable rather than a categorical variable. Additional methods which can’t be used to conduct classification analyses may be used for predictive analyses with continuous variables and vice-versa.
Unsupervised learning
Unsupervised learning is when the data miner task is to detect patterns based only on independent variables. It is generally presented more from an algorithmic fashion rather than from a purely statistical fashion. Well-known methods applied to marketing includes: (1) Market Basket Analysis and (2) Clustering.
Market Basket Analysis: Market basket analysis (also abbreviated as MBA to confuse you even more) is certainly one of the most known and easier task relating data mining and marketing. It is considered more as a typical marketing application rather than as a data mining method. It can be simplified as a simple Amazon recommendation algorithm showing as an association rule that “the probability that customers who bought item A also bought item B is 56%”. The classic urban legend about Market Basket Analysis is the “beer” and “diapers” association where a large supermarket chain, most people will say Walmart, did a Market Basket Analysis of customers’ buying habits and found an association between beer purchases and diapers purchases. It was theorized that the reason for this was that fathers were stopping off at Walmart to buy diapers for their babies, and since they could no longer go to bars and pubs as often as before, they would buy beer as well. As a result of this finding, the supermarket chain managers have placed the diapers next to the beer in the aisles, resulting in increased sales for both products.
Clustering: The method of Clustering is defined as the assignment of a set of observations (customers) in subsets (clusters) where customers in a cluster are similar to each other while they are different from other customers in other clusters. Clustering is often used in marketing for segmentation tasks. However, even though segmentation may be achieved through “clustering”, more modern supervised methods such as Bayesian Mixture Models, which I must say are not really part of the data mining field, are used by the few practitioners who can actually understand how to program this method (this is one method I am programming these days). For more about segmentation, I would refer anyone to the book “Market Segmentation: Conceptual and Methodological Foundations” by Michel Wedel and Wagner A. Kamakura, both are professors and well-known authorities on the topic.
Some Top References
I must say without a doubt that the best book I know about data mining is surely “Elements of Statistical Learning” by Stanford Professors Trevor Hastie, Robert Tibshirani, and Jerome H. Friedman which covers broadly and nearly every type of methods you can use to conduct data mining tasks. However I must admit that this book has a focus on the statistics behind the methods (but it’s extremely clear) rather than on the software tools (No, it’s not a cookbook) you could use to conduct these analyses, and it may also lack of marketing applications for a marketer. Furthermore, to get some updates about the data mining world, KD Nuggets, administered by Gregory Piatetsky-Shapiro, is actually THE reference for the data mining world.
Some Top Software
Here is a description of some software I would recommend for data mining tasks, feel free to propose your own software in the comments section:
1. R: R is actually my favorite software. I have been using the software for mainly all of my statistics-related tasks for the last 2 years. Its free, open source, it has an extensive and very knowledgeable community, it’s extremely intuitive and it can be learned more easily if you have knowledge of software such as C++, Python and/or GAUSS. Furthermore, there are a lot of useful packages available to facilitate the coding. However, I must say that compared to C++ or SAS, sometimes R can be slow for data mining tasks involving a heavy load of data.
2. rattle: rattle, which stands for the R Analytical Tool To Learn Easily, is a “point and click” data mining interface related to R and developed by Graham Williams of Togaware. Frankly, I must admit that this software rocks even though I generally don’t like “point and click” software. It’s extremely complete and quite easy to use.
3. SAS Enterprise Miner: SAS Enterprise Miner, a module in SAS, was the first software I used for performing data mining tasks. It is extremely fast and user-friendly. However, I must admit that it reminds me software like Amos, now included in PASW (formerly SPSS) for Structural Equation Modeling (SEM) tasks, where you move the “little truck” to build your model and don’t really understand what you’re doing at the end of the day. Furthermore, it costs a lot but to my knowledge, SAS is the only software platform integrating data mining tasks with web analytics and social media analytics.
4. RapidMiner: RapidMiner formerly known as YALE (Yet Another Learning Environment) is considered by multiple data miner as THE software to use to conduct data mining tasks. Similarly to R, the software is open source as well as free of charge for the “Community” version. I haven’t made the switch from R to RapidMiner yet and I am currently testing the software in depth.
5. Salford Systems: I must confess that I never used Salford Systems software but know them by reputation, thus, I can’t have a clear personal opinion on the software. However, statisticians working at Salford Systems are presenting workshops on data mining for the next Joint Statistical Meeting (JSM) in Miami at the end of July 2011 which I might attend.
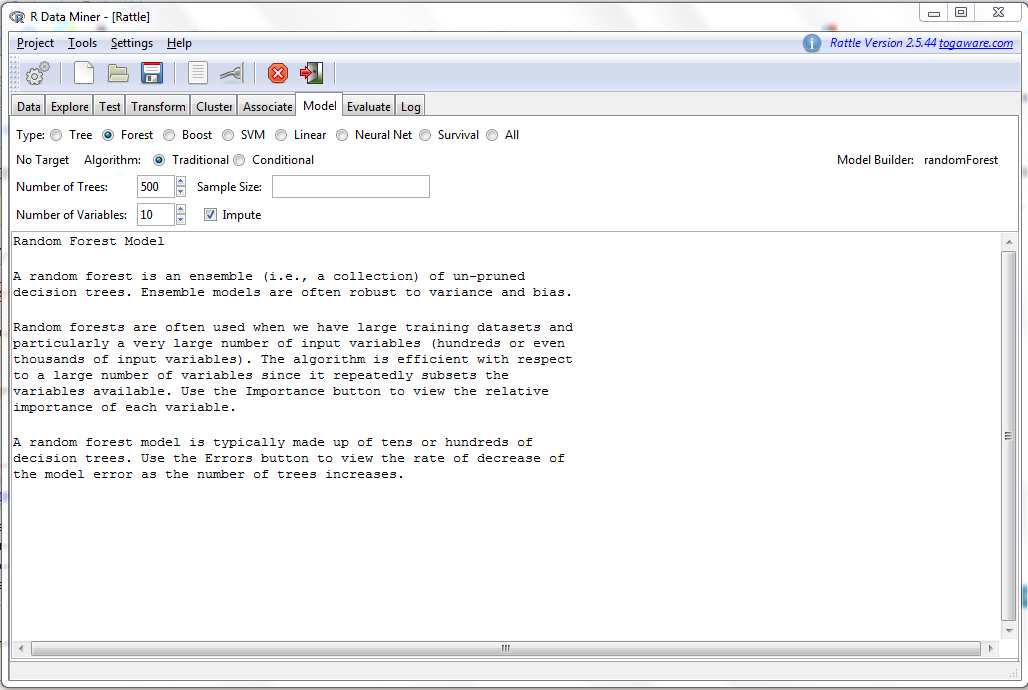
Waiting time and Conclusion
Whatever the software you’re using, data mining-related tasks will always be demanding in terms of your computer memory. Data Mining in marketing and business intelligence and more broadly KDD is an art that requires strong statistical skills but also a great comprehension of marketing problems. So when you’re waiting for your data mining computations, feel free to come by and read my other cool posts on your other computer! In anyways, enjoy data mining and as one of my friend would say “show some respect to the machine”, but even more to the data miner!
Cheers,
Jean-Francois Belisle